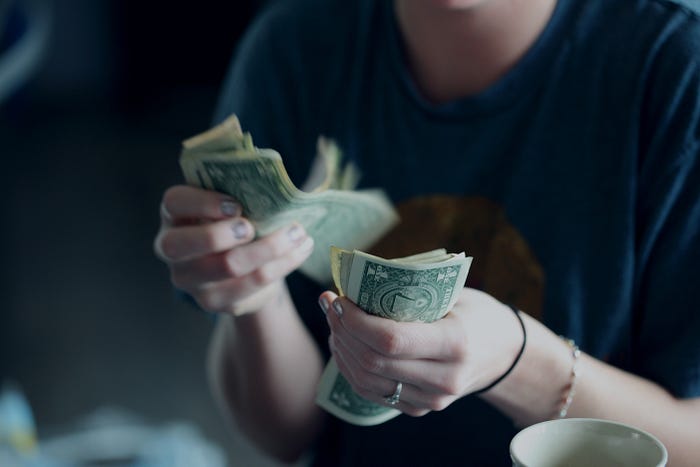
Estimating the $ of a Security Incident.
Using growth metrics to help forecast a bad day.
Let’s respond to a question from your boss:
How bad would it be if we were breached?
- Could you do better than saying: “Uh… real bad?”
- Instead, would you gather data to support your analysis?
- Do you want to develop a forecast of revenue impact to your organization?
Let’s prepare to answer your boss by embracing approximation. So long as you have access to some product and revenue data… you can quantify and forecast revenue impact to the organization that results of a future breach.
This essay will use financial data from a SaaS business that currently sees about $1.5M in Monthly Recurring Revenue (MRR) from their customers.
This company has been breached before. We’ll be doing subjective analysis of a future breach with this historical breach data as a useful reference point.
We’ll use the simple risk measurement description of credible interval forecasting to estimate the impact of a subsequent breach with 90% probability of its consequences to revenue.
Victim company name is excluded from the essay to keep the focus on analysis.
The Executive Summary
This company could see the following hits to MRR if a similar incident were to occur again:
- $8k-$50k of MRR in a well handled incident.
- $14k-$150k of MRR in a not so well handled incident.
- $50k-$400k of MRR if incident handling goes horribly wrong.
I would expect the rarest cases (top and bottom 5%) to go outside these dollar values, and the most likely outcome (90%) to fall within those dollar values.
Put another way: I expect to be surprised by a bad outcome in only 5% of cases where I have similar information. Let’s discuss how I formulated that opinion.
Analyzing user and revenue growth metrics.
We’ll model our forecast after customer and revenue growth metrics language and estimate how much Monthly Recurring Revenue (MRR) a company could lose as a result of a breach.
This SaaS business describes their customer growth and monthly revenue with the following categories:
- New: Brand new customers that subscribed for the first time.
- Retained: Customers who are… still customers!
- Expanded: Customer that starting paying more after an upgrade.
- Contracted: Customers who have downgraded and pay less.
- Churned: Customers who have stopped paying.
- Reactivated: Customer who once churned. They’re back!
This gives us some language to understand revenue patterns if we want to approximate how much a breach will impact them.
How do active customers typically fluctuate?
This helps inform us what normal customer activity looks like. Here’s the typical active customer growth for this company on any given day over the course of a month. We need to know what normal looks like to approximate how any major event could influence these numbers.

This company lost 151 net customers on their worst day of a recent month. They added 50 net customers on their best day.
This company has also seen anywhere between:
- 37–157 “new” customers daily
- 9–35 customers “reactivate” daily
- 74–291 customers “churn” daily
Now we’re creating some mental bounds to estimate with. It seems improbable that this company will lose millions of customers in a minute, for instance. It’s also not growing customers so aggressively that a major event would still end up with overall growth.
Note: You might notice that this company is “losing” customers slowly. However, they seem to be transitioning towards larger customers, as you’ll see below.
How drastically does their MRR fluctuate?
You can see Monthly Recurring Revenue (MRR) fluctuate daily as customers join and churn, expand and contract. Solely tracking active customer changes might not work reflect the reality of how revenue fluctuates. We should get a sense of how this looks, too.
Currently, this company has about $1.5M in MRR. Here’s a recent month showing how this MRR can fluctuate from day to day.

We can see that MRR can move by a couple thousand dollars in either direction on a typical day. The company is generally growing in MRR over the course of the month. This knowledge informs our baseline of what normal looks like.
How much do customers fluctuate in value?
A revenue impact from a breach could vary greatly depending on the types of customers we lose. We need to understand how harmful losing some customers over others would be.
A majority (>85%) of this business’ customers generate between $10-$15 MRR from a mix of annual or monthly plans. The top paying customers generate up to $600 MRR, but large customers make up less than 15% of overall customers. Some rules of thumb:
- A single churn of a large customer could result in a loss of ~$600 MRR.
- A single churn of a basic customer could result in a loss of $10–15 MRR.
Losing a group of customers could include some uncertain amount of either group. If a given incident biased toward larger customers, it could quickly balloon the revenue impact as a result. For instance, if large customers were somehow targeted in a breach.
Let’s revisit their previous “bad day”.
It was many years ago when this company saw its first public security incident. This is useful for us as a close reference to approximate a similar incident in the future.
After their breach:
- Churn doubled and new customers took a small hit the day after this company’s breach.
- Their bad day saw about a
0.89%
loss to MRR over two days. - After two days, growth and revenue trends went back to normal.
It was a crisis for the company but it was far from devastating, and here’s why:
They handled their breach really well.
The event was widely publicized. Their mitigation required customers of the victim to reconfigure their product to continue service.
The company was transparent throughout the response and their customers were highly supportive throughout the incident.
Growth metrics bounced back just a day later returned to normal trends.
Serious business risk was largely mitigated. Additionally, the data they stored was not personally sensitive. It didn’t trigger any sort of sustained public outrage or regulatory action.
Doing this at your org? Do you, or others, have reference losses?
Let’s estimate a *new* bad day.
Consider if a similar incident were to happen to this company… again!
Would it go just as well as the last one? We can’t be certain. It could go even better, but it could also go far worse. Let’s not assume our breaches are well handled.
The following variables are possible:
- You might be requiring your customers to take more painful actions.
- You might be offline for a longer duration of the incident response.
- There might be longer press coverage of your incident.
- There might be higher, angrier piles of customer inquiries.
- Competitors might be more successful luring customers over.
Here are three future incident forecasts:
I’ll estimate the impact of a similar “bad day” for this company going forward. These estimations produced the intervals at the beginning of the essay.
We’ll discuss three example incident response outcomes. We’ll use some of the applicable methods mentioned here to approximate losses.
In all of the three following “example” incidents, similar to the last one.
- Customers have varying hurdles to regain service.
- There’s varying success in responding to ticket volume.
- There’s an outage, to varying lengths.
- Publicity is varying in duration and criticism.
Remember the previous incident. Churn doubled, new business took a small hit, and MRR dropped by 0.89%
. There would be an MRR loss of about $15k should this percentage impact be naively applied to today’s MRR.
A terribly handled incident: There’s a full day outage. Customers have no idea how to recover. Communications are delayed, criticized, misleading, and botched. Headline coverage in tech communities and blogs have spiraled out for 3+ days. You’ve lost control the message and customers are talking to each other more than they’re talking to you. Competitors have enough time to capitalize on their frustration.
There is a 90% chance of 600 to 15000 customer losses and a 90% chance of $50k-$400k of losses to MRR.
A pretty bad incident: There’s a half day outage. Communications are reasonable. Media coverage is limited to a day or two, and is mostly tracking progress.
There is a 90% chance of 210 to 800 customer losses and a 90% chance of $14k-$150k of losses to MRR.
A well handled incident: There are hours of outage. Customer mitigation is cut and dry. Customer communications are proactive and informative. Support crushes it on responsiveness. Media and community response is positive and commentary support you. Very similar to previous incident.
There is a 90% chance of 70 to 600 customer losses and a 90% chance of $8k-$50k of losses to MRR.
What’s up with these numbers?
I coded my belief of the potential impacts into a 90% credible interval using some brief review of the company’s historical data, some information about previous outages, and my knowledge of how incidents are handled. These are forecasts. They are subjective, quantified, and testable just like a weather forecast.
Forecasts usually contain some amount of uncertainty, making them wrong by definition. This is really valuable. We should be open to being wrong so that we can tune our beliefs about risks into being less wrong and continuously improving.
Why are they always wrong? An uncertain forecast leaves the possibility that a true value can live outside the interval, or, within it. I expect to be surprised in only 10% of cases I forecast with 90% certainty, but I’m not totally certain (100%) of one case or the other.
No matter what happens, the forecast is either a little bit wrong, or really wrong. I will be least surprised if a future breach has an impact within the intervals I’ve created when incident response goes as described.
What influenced my forecast?
Here’s what was running through my mind while approximating:
- The previous incident is a hugely important data point. The “Good Response” will probably look similar in magnitude to the previous breach.
- However, the company has grown quite a bit since it’s previous incident. It has a larger audience and customers are spending more. I can’t copy the previous incident impact. Instead, I have to work with its current proportions, and reconsider the new value of a customer.
- I expanded and positioned an interval starting from percentage damages from the previous incident to compensate for these unknowns.
- The interval I approximated (90% of possible incidents) excludes the top (and bottom) 5% of incident response possibilities. If I wanted to include absolute extreme scenarios, I could very simply approach this again with 95–99% intervals in mind.
- A media cycle for most incidents will end in single digit days, without follow up. I know this from personal experience. This company seemed unlikely to have sustained headlines in a breach. This area also has a reference class available of how stocks are influenced after a breach, which also help approximate “length” of attention by observers.
- This company has absurdly loyal customers who were openly cheering for the company during the incident.
- This company is not supporting mission critical infrastructure for its customers. (A prolonged outage isn’t a @badthingsdaily tweet)
- If I didn’t have the reference incident, I would have probably widened the interval quite a bit from my current forecast. I was careful not to narrow it too much to overcompensate by having reference data.
- It’s quite difficult to determine if large customers would leave in greater proportion than their standard customers, so I have to account for that uncertainty with larger intervals than just an “average customer MRR”.
What can be said with this data?
Now we can make a casually defensible statement based on our research, similar to the introduction of this essay. This may be more effective than saying “Our breach would be hella bad!” and allows us to present a quantitative finding to leadership.
We can now discuss a potential breach with a dollar value with today’s knowledge:
If a similar incident were to be handled poorly, I think there is a 90% probability we could lose $12k–$400k in MRR.
If we could handle it as well as last time, it will look more like $8k-$50k of lost MRR.
If we’re asked to provide a more rigorous analysis, there are plenty of ways to do so.
Remember: the impact to revenue is a single area of impact measurement. There are plenty of other areas to consider: Employee time spent, ongoing costs as a result of regulation, and penance payments (as described in this book) are additional costs that you can consider as well.
I think our intuition is correct that security breaches are really expensive, and this essay doesn’t fully solve for that.
Instead, breaches are easier to interpret as a sum of many uncertain impacts when approached with methods like this. It takes some effort to tease out the different dimensions of cost that are involved with the true cost of a breach.
Conclusion
I’ve demonstrated a casually defensible forecast of breach impact to a real company’s revenue using approximation methods. This helps us tailor a measurement of breach impact to our specific organizations to help us find better efficiency in all aspects of risk management.
I’m really thankful that I’m able to review this company’s data. I am intentionally leaving the company name out to avoid penalizing them for being transparent. The lessons from their experience are more important than revisiting a years old incident. Thanks!
Ryan McGeehan writes about security on scrty.io